LLMs: Redefining Customer Support
The integration of Large Language Models (LLMs) into customer support has ushered in a paradigm shift, redefining efficiency, scalability, and personalization in customer interactions. By 2025, 80% of customer service organizations are projected to adopt generative AI technologies like LLMs to enhance agent productivity and satisfaction. These models enable 24/7 multilingual support, automate routine tasks, and deliver hyper-personalized responses, reducing operational costs by up to 50% in some cases. However, challenges such as hallucination risks and the need for robust evaluation frameworks persist, requiring ongoing innovation in areas like Retrieval-Augmented Generation (RAG) and sentiment analysis. As LLMs evolve to incorporate emotional intelligence and predictive capabilities, they are poised to transition customer support from a cost center to a strategic driver of loyalty and revenue.
Evolution of Customer Support in the AI Era
From Human-Centric to AI-Driven Interactions the customer support landscape has evolved from face-to-face interactions and phone-based systems to digital platforms powered by LLMs. Traditional models relied on human agents to handle repetitive inquiries, leading to bottlenecks during peak demand and inconsistent service quality. LLMs address these limitations by automating up to 75% of routine queries, as demonstrated by startups that slashed response times while doubling customer satisfaction scores. By processing natural language with human-like fluency, these models resolve issues ranging from billing disputes to technical troubleshooting without human intervention.
The Role of Contextual Understanding
Modern LLMs leverage large context windows to retain conversation history, ensuring customers no longer need to repeat information across sessions. For instance, Swiggy’s food delivery chatbot uses this capability to track orders, estimate delivery times, and proactively notify users of delays. Similarly, Netflix’s recommendation system analyzes viewing patterns to suggest content, reducing the need for manual search queries. This contextual awareness is further enhanced by function calling, where LLMs integrate with backend systems to retrieve real-time data (e.g., order status, account details).
Core Applications of LLMs in Customer Support
Automation of Routine Tasks
- Intelligent Chatbots and Ticket Management: LLM-powered chatbots handle 60–70% of inbound queries in sectors like e-commerce and SaaS, resolving issues in seconds rather than hours. For example, Lenovo’s AI-driven Premier Support system reduced average handle time by 20% by automating post-call summaries and ticket categorization.
- Knowledge Base Generation and Maintenance: LLMs dynamically update FAQs and troubleshooting guides by analyzing customer interactions, as demonstrated by eBay’s LiLiuM model and eGain’s AI Knowledge Hub.
Personalization at Scale
- Dynamic Customer Profiling: By synthesizing purchase history, browsing behavior, and communication preferences, LLMs deliver hyper-personalized support akin to Amazon-level personalization. Examples include Spotify’s playlist recommendations and tailored financial advice from banking LLMs.
- Predictive Support and Proactive Engagement: Advanced LLMs anticipate issues before they arise, enabling telecom providers and companies like Alibaba to notify users of potential problems preemptively.
Breaking Language and Geographic Barriers
Real-Time Multilingual Support
LLMs like those deployed by eBay and Alibaba provide instant translation across 100+ languages, eliminating the need for regional support teams. Lenovo’s omnichannel chatbot handles multiple languages with high proficiency and customer satisfaction.
Cultural Localization
Beyond translation, LLMs adapt responses to cultural norms. For instance, they offer formal greetings during cultural events or concise, honorific-laden replies based on local customs, reducing miscommunication-related escalations.
Challenges and Mitigation Strategies
Hallucination and Accuracy Risks
Despite advancements, LLMs occasionally generate plausible-sounding but incorrect answers. Frameworks like Helpshift’s Retrieval-Augmented Generation (RAG) combat this by grounding responses in verified knowledge bases, significantly reducing hallucinations. Evaluation metrics ensure outputs align with organizational guidelines.
Ethical and Bias Concerns
Bias detection algorithms audit LLM outputs for demographic skews. Regular fine-tuning on diverse datasets has mitigated significant biases, ensuring fairness in sensitive applications such as finance and healthcare.
Future Directions and Industry Implications
Integration with Emerging Technologies
The convergence of LLMs with speech recognition and AR/VR technologies will enable immersive support experiences. For example, automotive companies are testing AI-powered virtual mechanics that guide users through repairs via augmented reality overlays.
Emotional Intelligence and LBMs
Large Behavior Models (LBMs) trained on human behavioral cues are enabling chatbots to mimic empathy authentically, potentially increasing customer retention by detecting subtle frustration cues and escalating issues preemptively.
Economic Impact
By 2026, AI-driven support is projected to save businesses $1.2 trillion annually. Evidence suggests that reducing human agent involvement while doubling efficiency can significantly boost profit margins.
Conclusion
LLMs have transitioned customer support from a reactive cost center to a proactive growth engine. They enable hyper-personalized, instantaneous, and multilingual service at scale, leading to substantial improvements in customer satisfaction. As emotional intelligence and predictive capabilities mature, LLMs will unlock unprecedented levels of customer loyalty—positioning AI not as a human replacement, but as a strategic collaborator in decision-making. Organizations that embrace this transformation will lead in the age of AI-driven customer experience.
About the Author
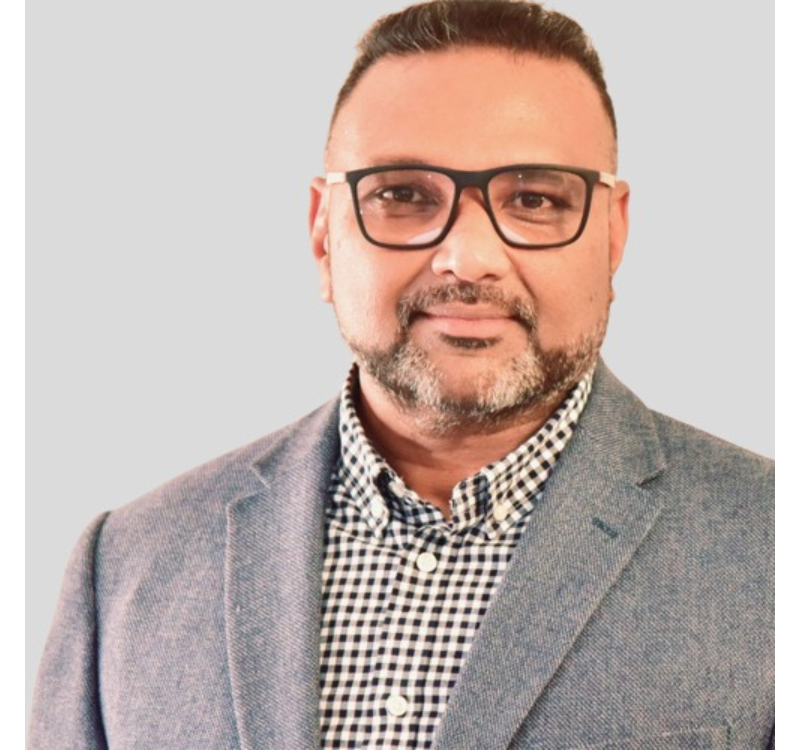
Rejith Krishnan
Rejith Krishnan is the Founder and CEO of lowtouch.ai, a platform dedicated to empowering enterprises with private, no-code AI agents. With expertise in Site Reliability Engineering (SRE), Kubernetes, and AI systems architecture, he is passionate about simplifying the adoption of AI-driven automation to transform business operations.
Rejith specializes in deploying Large Language Models (LLMs) and building intelligent agents that automate workflows, enhance customer experiences, and optimize IT processes, all while ensuring data privacy and security. His mission is to help businesses unlock the full potential of enterprise AI with seamless, scalable, and secure solutions that fit their unique needs.
“`