Comparing Pricing: GPT-4o Mini vs. Grok-2
GPT-4o mini and Grok-2 represent two competing offerings in the cost-efficient AI model space, though with dramatically different pricing structures. This comprehensive analysis examines the pricing models of these two language models, their relative cost efficiency, and the implications for developers and organizations considering either solution for their AI applications.
Pricing Structure Comparison
OpenAI’s GPT-4o mini and xAI’s Grok-2 employ similar pricing mechanisms by charging separately for input tokens (text sent to the model) and output tokens (text generated by the model), yet the actual costs differ significantly.
GPT-4o mini Pricing
- Input tokens: $0.15 per million tokens
- Output tokens: $0.60 per million tokens
This model offers an order of magnitude lower cost than previous frontier models and is more than 60% cheaper than GPT-3.5 Turbo, making it ideal for high-volume applications and real-time interactions.
Grok-2 Pricing
- Input tokens: $5.00 per million tokens
- Output tokens: $15.00 per million tokens
These rates place Grok-2 at a substantially higher price point, positioning it as a premium option relative to GPT-4o mini.
Direct Cost Comparison
For 1 million tokens:
- Input: GPT-4o mini: $0.15 vs. Grok-2: $5.00 (33.3× more expensive)
- Output: GPT-4o mini: $0.60 vs. Grok-2: $15.00 (25× more expensive)
Context Windows and Capabilities
Both models offer similar technical specifications in terms of context windows, each supporting a 128K token context. This enables both to process large amounts of text in a single request.
Performance Considerations
GPT-4o mini Performance
- Scores 82% on MMLU (Massive Multitask Language Understanding)
- Outperforms GPT-4 on chat preferences in the LMSYS leaderboard
- Supports text and vision inputs, with future support for video and audio
- Knowledge cutoff: October 2023
Grok-2 Performance
- Scores 87.5% on MMLU using 0-shot Chain of Thought
- Outperforms models like Claude 3.5 Sonnet and GPT-4-Turbo on the LMSYS leaderboard
- Features advanced capabilities in text and vision understanding
- Knowledge cutoff: Uncertain
Cost Implications for Different Use Cases
The dramatic price difference creates distinct use cases:
- GPT-4o mini: Ideal for high-volume token processing, chatbots, customer service, parallel model calls, and processing large documents or code bases. Perfect for cost-sensitive deployments.
- Grok-2: May be preferred for applications demanding advanced reasoning capabilities, complex tasks, or for users already integrated with the xAI ecosystem.
Comparative Context in the LLM Market
For additional context:
- GPT-4o (larger model): $2.50 per million input tokens and $10 per million output tokens
- GPT-4.5 Preview: $75 per million input tokens and $150 per million output tokens
- GPT-3.5 Turbo: $0.50 per million input tokens and $1.50 per million output tokens
- o1: $15 per million input tokens and $60 per million output tokens
- o1-mini: $1.10 per million input tokens and $4.40 per million output tokens
This positions GPT-4o mini as one of the most affordable options, while Grok-2 sits in a middle tier between basic and premium offerings.
Conclusion
The pricing comparison reveals a stark contrast in cost structures despite similar technical specifications. GPT-4o mini, at $0.15 per million input tokens and $0.60 per million output tokens, is among the most affordable models available. In contrast, Grok-2, with pricing of $5.00 per million input tokens and $15.00 per million output tokens, occupies a significantly higher price point. For cost-sensitive applications and high-volume use cases, GPT-4o mini offers compelling value. However, for scenarios that demand Grok-2’s advanced performance characteristics, its premium pricing may be justified. Organizations must carefully assess their specific needs and usage volumes when choosing between these models.
About the Author
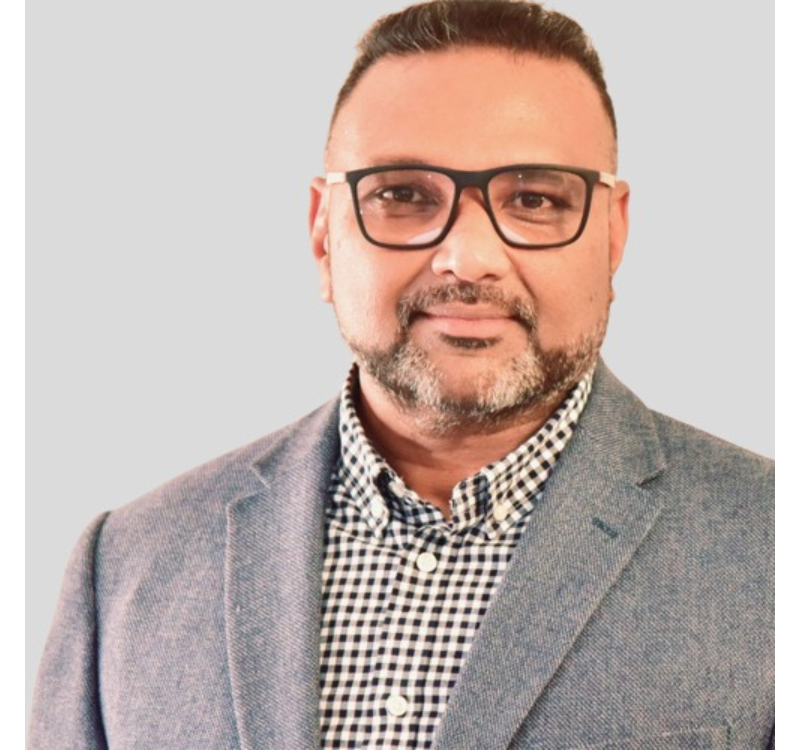
Rejith Krishnan
Rejith Krishnan is the Founder and CEO of lowtouch.ai, a platform dedicated to empowering enterprises with private, no-code AI agents. With expertise in Site Reliability Engineering (SRE), Kubernetes, and AI systems architecture, he is passionate about simplifying the adoption of AI-driven automation to transform business operations.
Rejith specializes in deploying Large Language Models (LLMs) and building intelligent agents that automate workflows, enhance customer experiences, and optimize IT processes, all while ensuring data privacy and security. His mission is to help businesses unlock the full potential of enterprise AI with seamless, scalable, and secure solutions that fit their unique needs.