Introduction
This article explores how financial institutions can enhance Enterprise Intelligence with AI and advanced models like LLMs and LSTMs, highlighting a real-world case where a large bank utilized AI to identify breaking points, single points of failure, and troubleshoot root causes, ultimately strengthening operational resilience.
In today’s rapidly evolving financial landscape, large enterprises constantly face the challenge of maintaining operational efficiency while meeting ever-growing customer demands. Artificial Intelligence (AI), particularly Large Language Models (LLMs) and Long Short-Term Memory (LSTM) networks, has emerged as a transformative technology enabling organizations to optimize processes, enhance system reliability, and deliver superior customer experiences.
The Impact of AI on Large Enterprises
Enhancing Operational Efficiency
Automating routine tasks reduces manual effort, minimizes errors, and accelerates service delivery.
Improving Decision-Making with Enterprise Intelligence
AI-driven analytics provide deep insights into data, enabling proactive strategies and informed decisions.
Elevating Customer Experience
Personalized services and faster response times improve customer satisfaction and loyalty.
Strengthening Security
Advanced algorithms detect and mitigate risks, protecting sensitive financial data from threats.
Leveraging AI Models like LLMs and LSTMs in Financial Enterprises
Financial institutions handle vast amounts of data and complex infrastructures. Successfully leveraging AI requires:
Understanding Key AI Models
- Large Language Models (LLMs)
- Function: LLMs like GPT-3 understand and generate human-like text, enabling advanced natural language processing tasks.
- Benefits: Enhance customer service through chatbots, automate report generation, and analyze unstructured data.
- Long Short-Term Memory (LSTM) Networks
- Function: LSTMs are recurrent neural networks that excel at learning sequences, useful for time-series forecasting and anomaly detection.
- Benefits: Ideal for predictive maintenance and enterprise systems monitoring.
- AIOps (Artificial Intelligence for IT Operations)
- Function: AI automates IT operations, enhancing efficiency and reducing human intervention.
- Benefits: Detects system anomalies, predicts outages, and automates problem resolution.
- Site Reliability Engineering (SRE)
- Function: Applies engineering principles to IT operations, creating scalable and reliable systems.
- Benefits: Improves system reliability and reduces downtime.
Real-World Use Cases and Insights
Case Study 1:
Enhancing Enterprise Intelligence with AI
A large financial institution struggled with frequent system outages and prolonged resolution times, affecting customer experience and operational efficiency.
Solution:
- AIOps with LSTM Models: The bank integrated AIOps platforms using LSTM networks to monitor IT infrastructure in real-time and detect anomalies.
- Breaking Points Identified: AI models pinpointed single points of failure and potential breaking points before disruptions occurred.
- Root Cause Analysis with LLMs: LLMs rapidly interpreted unstructured data like error logs, identifying the root causes of issues such as authentication failures.
Results:
- Reduced Downtime: System outages dropped by 40%, improving service availability.
- Faster Issue Resolution: Incident resolution times decreased by 50%.
- Improved Customer Trust: Enhanced system reliability boosted customer confidence.
Case Study 2:
Leveraging SRE and Anomaly Detection for Reliability
Solution:
- Real-Time Monitoring: Implemented LSTM models to continuously monitor performance and detect anomalies.
- Proactive Issue Identification: SRE teams received alerts, preventing issues from impacting customers.
Results:
- Increased System Stability: Early detection prevented failures.
- Optimized Resource Allocation: AI insights improved capacity planning.
Case Study 3:
Transforming Customer Service with Help Desk Automation
Solution:
- AI-Powered Chatbots: Virtual assistants handled customer queries, improving response times.
- Intelligent Routing: Complex issues were automatically escalated to human agents.
Results:
- Reduced Response Times: Average response times decreased by 60%.
- Cost Savings: Automation reduced the workload on human agents.
- Enhanced Customer Satisfaction: Improved efficiency led to higher satisfaction ratings.
Insights on Implementing AI Solutions
The Role of AI in AIOps and Enterprise Intelligence
- Automation of Routine Tasks: Frees up IT staff for strategic work.
- Predictive Maintenance with LSTM Models: Preemptive actions prevent system failures.
- Enhanced Visibility: AI provides comprehensive insights into IT environments.
- Enterprise Intelligence: AI and LLMs extract insights from unstructured data, driving strategy.
Conclusion
AI is no longer a futuristic concept but a necessity for large financial enterprises aiming to stay competitive and efficient. By embracing AI technologies like LLMs, LSTMs, AIOps, and help desk automation, financial institutions can transform operations, improve reliability, and deliver exceptional customer experiences.
About the Author
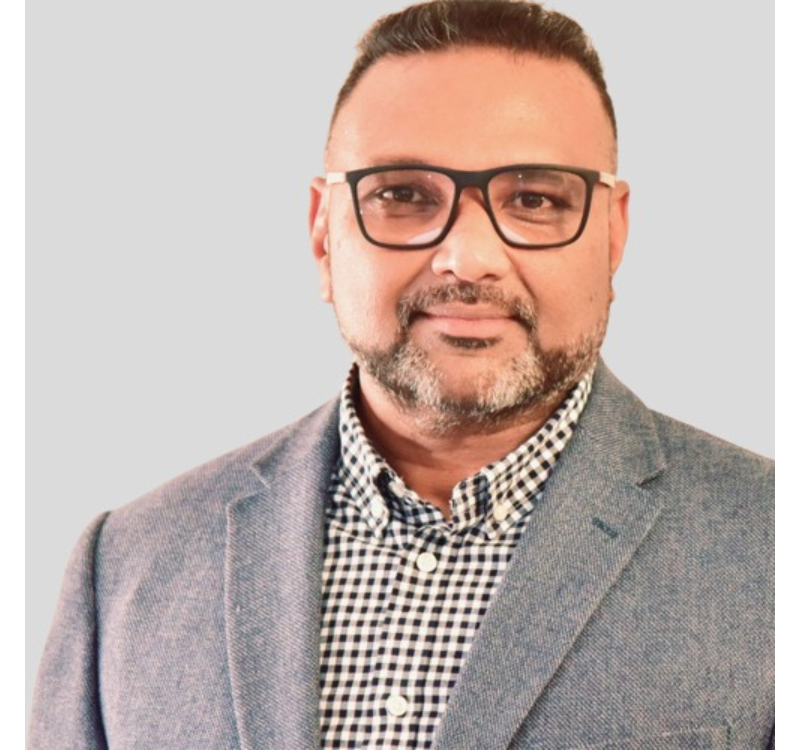
Rejith Krishnan
Rejith Krishnan is the Founder and CEO of lowtouch.ai, a platform dedicated to empowering enterprises with private, no-code AI agents. With expertise in Site Reliability Engineering (SRE), Kubernetes, and AI systems architecture, he is passionate about simplifying the adoption of AI-driven automation to transform business operations.
Rejith specializes in deploying Large Language Models (LLMs) and building intelligent agents that automate workflows, enhance customer experiences, and optimize IT processes, all while ensuring data privacy and security. His mission is to help businesses unlock the full potential of enterprise AI with seamless, scalable, and secure solutions that fit their unique needs.