Introduction
In the ever-evolving world of banking, financial institutions are continuously seeking innovative ways to enhance operational efficiency, reduce costs, and improve customer service. As more processes move online and customer expectations for personalized service grow, the need for data-driven decision-making and automation has never been more pressing. This project for a leading financial institution demonstrates how the integration of AI technology can transform core banking operations and customer interactions, yielding significant improvements in efficiency and service quality.
This article explores the objectives, challenges, and outcomes of the project, showcasing how AI can drive meaningful change in the banking sector, from automating routine tasks to providing more personalized customer experiences.
The Challenge
This financial institution, like many in the industry, was facing several critical challenges:
- Manual, Time-Consuming Processes: Core banking operations, such as loan processing, document verification, and customer support, were heavily reliant on manual input, leading to inefficiencies and delays in service delivery.
- Data Overload: The bank handled vast amounts of transactional and customer data daily. However, extracting actionable insights from this data required significant manual effort and expertise, limiting the bank’s ability to make data-driven decisions in real time.
- Customer Expectations: Customers were increasingly demanding personalized services, faster response times, and a seamless experience across both online and offline channels. The bank’s existing systems lacked the ability to provide personalized recommendations or predictive insights into customer needs.
- Operational Costs: Rising operational costs, coupled with the complexity of managing both traditional and digital banking systems, necessitated a more efficient, scalable approach to resource management.
The bank knew that leveraging AI technology was key to addressing these issues, but they required a partner who could guide the integration of AI into their existing infrastructure without disrupting current operations. That’s where the AI architecture team stepped in.
The Objective
The objective of this project was to design and implement an AI-driven solution that would:
- Enhance operational efficiency by automating key processes such as loan approvals, fraud detection, and customer service tasks.
- Improve data utilization through predictive analysis and machine learning, enabling the bank to make real-time, data-driven decisions.
- Deliver personalized customer experiences by leveraging AI models to analyze customer behavior and provide tailored financial advice and services.
- Reduce operational costs by optimizing resource usage, automating routine tasks, and increasing staff productivity.
The project’s ultimate goal was to enable the bank to maintain its competitive edge in the rapidly changing financial landscape, while also improving customer satisfaction.
The Solution: Leveraging AI to Transform Banking Operations
To meet these objectives, the AI team designed and implemented a comprehensive AI solution, leveraging machine learning models, predictive analytics, and natural language processing (NLP) to automate processes and enhance decision-making.
Automating Routine Banking Operations
One of the key areas where AI made an immediate impact was in the automation of routine banking processes. By utilizing machine learning models, the team was able to streamline operations that had previously been manual and time-consuming.
- Loan Processing: The AI solution was able to automate much of the loan processing pipeline. From initial application review to final approval, machine learning models could assess credit risk, analyze customer profiles, and recommend approval or rejection, all in a fraction of the time it would take human agents. This not only sped up the process but also reduced the likelihood of human error.
- Document Verification: Using NLP and computer vision, the AI system was able to scan and verify documents such as identification and income statements in real time. This helped reduce the time it took to process documents and ensured greater accuracy, leading to fewer rejected applications.
- Fraud Detection: By analyzing transaction patterns, AI models were able to flag potentially fraudulent activities. The system could detect anomalies in customer behavior—such as unusual transaction volumes, patterns, or locations—and alert bank staff to investigate further. This real-time fraud detection capability greatly reduced the bank’s exposure to financial risk.
Predictive Analytics for Enhanced Decision-Making
AI-driven predictive analytics were used to empower the bank with real-time insights into customer behavior, financial trends, and operational performance.
- Customer Behavior Analysis: By analyzing transaction data, customer inquiries, and account histories, machine learning algorithms were able to predict customer needs, such as which customers were likely to apply for a loan or open a new account. This enabled the bank to proactively reach out with personalized offers or financial advice, improving customer engagement.
- Resource Allocation: Predictive analytics also played a key role in optimizing resource allocation. For example, the AI model could predict peak times for customer support and automatically adjust staffing levels to ensure that there were enough agents available to handle increased demand. This reduced wait times and improved customer satisfaction, while also cutting down on unnecessary staffing during slower periods.
Improving Customer Service with AI
AI-powered customer service solutions, such as chatbots and virtual assistants, were deployed to handle customer inquiries more efficiently. The goal was to provide faster responses and free up human agents to focus on more complex customer issues.
- 24/7 Virtual Assistance: The bank implemented an AI chatbot capable of answering customer questions, helping with account issues, and even guiding customers through loan applications. The chatbot was integrated with the bank’s knowledge base and could access customer data to provide personalized responses. This helped reduce the workload on customer support teams and ensured that customers could receive assistance around the clock.
- Intelligent Ticket Routing: When human assistance was required, the AI system automatically categorized and routed support tickets to the appropriate department or agent based on the nature of the inquiry. This reduced response times and ensured that customer issues were handled by the most qualified staff.
Reducing Operational Costs
By automating routine processes and improving the bank’s ability to make data-driven decisions, the AI solution led to a significant reduction in operational costs.
- Efficiency Gains: Automating tasks such as document verification, fraud detection, and customer support allowed the bank to operate with fewer manual resources. This not only cut down on staffing costs but also led to faster turnaround times and better customer satisfaction.
- Scalability: The AI system was designed to scale with the bank’s growing customer base. As demand increased, the AI models could handle more tasks without requiring additional infrastructure or personnel, allowing the bank to scale its services without corresponding increases in costs.
Results: A New Era of Efficiency and Personalization
The implementation of AI brought about dramatic improvements in both operational efficiency and customer satisfaction. Some of the key results included:
- 50% reduction in loan processing times: By automating much of the loan approval process, the bank was able to process applications in half the time it previously took.
- 40% improvement in customer support efficiency: The introduction of AI-powered chatbots and intelligent ticket routing led to faster response times and reduced wait times for customers.
- 30% reduction in operational costs: Automating routine tasks and optimizing resource allocation allowed the bank to operate more efficiently, cutting operational costs by nearly one-third.
- Enhanced fraud detection: The AI system’s real-time fraud detection capabilities reduced the bank’s exposure to fraudulent activities, providing a more secure banking environment for customers.
Conclusion
This project showcases how AI can transform core banking operations, enabling financial institutions to become more efficient, reduce costs, and provide personalized, data-driven services. By automating routine tasks, improving fraud detection, and enhancing customer service, AI allows banks to stay competitive in an increasingly digital world.
For any financial institution looking to improve its operational efficiency and customer service, the integration of AI-driven solutions presents a compelling opportunity to drive meaningful change and unlock new levels of performance.
About the Author
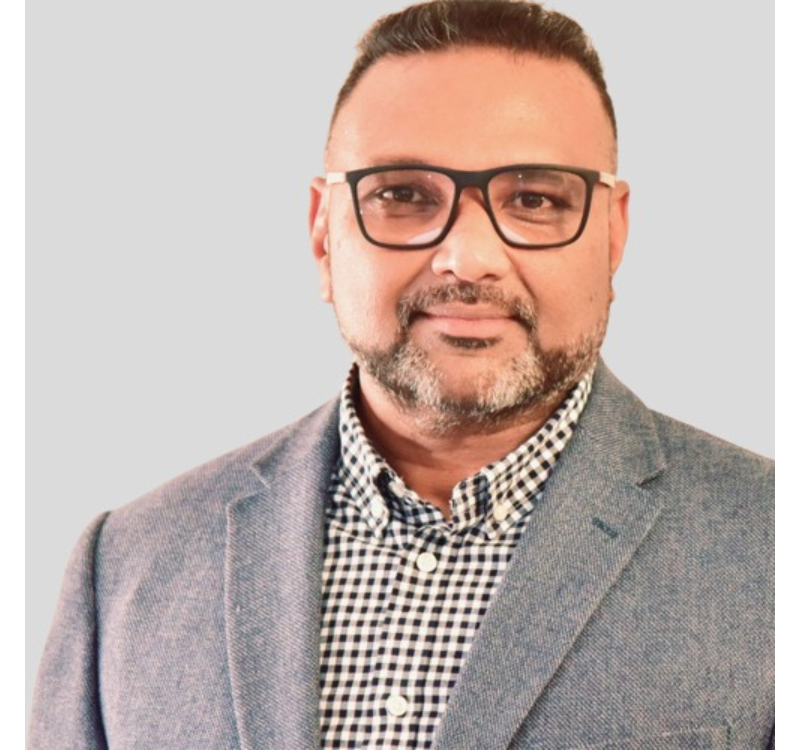
Rejith Krishnan
Rejith Krishnan is the co-founder and CEO of CloudControl, the parent company of lowtouch.ai. With a passion for simplifying AI-driven cloud services, Rejith is an expert in SRE (Site Reliability Engineering) and Kubernetes, constantly driving innovation in the field of enterprise AI solutions. His leadership at CloudControl has helped businesses integrate advanced AI technologies with ease, making them more efficient and scalable. Outside of work, Rejith enjoys spending time with his two sons and engaging in outdoor activities like hiking and kayaking.