Optimizing Cloud Total Cost of Ownership (TCO) Using AI: The Key to Smarter Cloud Spending
In today’s digital age, cloud computing has become an indispensable part of enterprise IT strategy. This brings scalability, flexibility, and access to cutting-edge technologies. But it also introduces a new challenge of managing the Total Cost of Ownership (TCO). With the complexity of cloud pricing models, the intricacies of service catalogs, and the ever-changing nature of discounts, enterprises often face unexpectedly high cloud bills. Cloud cost optimization with AI could be the solution!
This white paper explores how leveraging Generative AI (GenAI) and Machine Learning (ML) can transform cloud cost management, helping enterprises optimize their cloud spending. By analyzing service usage patterns, pricing models, and deployment strategies, AI-powered solutions can deliver significant cost savings—often 30-40% within just three months.
The Challenge of Managing Cloud TCO
Enterprises shifting to cloud-based infrastructure often encounter several challenges related to cost management:
- Complexity of Cloud Pricing Sheets: Cloud providers like AWS, Azure, and Google Cloud offer detailed pricing sheets that are difficult to decipher. These sheets include various pricing models—on-demand, reserved instances, spot pricing—each with different cost implications. The sheer volume of options can overwhelm even seasoned IT professionals.
- Cloud Bills Are Complex and Unforgiving: Cloud bills are notoriously difficult to understand. Often consisting of thousands of line items that detail usage across different services, regions, and accounts. The intricacies of billing practices make it challenging to identify areas where costs can be reduced without sacrificing service quality.
- Complex Service Catalogs and Dependencies: The vast number of services and features in cloud service catalogs & their dependencies, make it difficult to find the most cost-effective way to use cloud resources. A single service may rely on multiple underlying resources. This can create a ripple effect on costs.
- Impact on the Bottom Line: For enterprises with significant cloud investments, these challenges can lead to wasted spending, unoptimized deployments, and inflated TCO. As cloud usage grows, so does the risk of cost inefficiencies, potentially impacting profitability.
The Solution: Using GenAI and ML to Optimize Cloud TCO&amp;amp;amp;amp;amp;amp;amp;amp;amp;amp;amp;amp;amp;amp;amp;amp;amp;amp;amp;amp;amp;amp;amp;amp;amp;amp;amp;amp;lt;/h3></h3>
Analyzing C
loud Service Usage Month to Month</strong></h4>
<p>
By leveraging AI models to analyze cloud usage patterns on a month-to-month basis, enterprises can identify trends, spikes, and anomalies in service consumption. GenAI and ML algorithms can process historical usage data and correlate it with business events, helping enterprises understand the drivers behind cloud costs.
- Pattern Recognition: AI can detect such as daily or seasonal peaks and offer insights into how workloads are being utilized. This information can help companies make informed decisions about resource allocation.
- Anomaly Detection: AI models can detect unusual usage patterns that may indicate inefficiencies. Identifying idle resources, unused storage, or over-provisioned instances and addressing anomalies early can prevent unnecessary spending.
Matching Service Usage Against Cloud Providers’ Pricing Models
AI-driven optimization tools can map an enterprise’s cloud usage to the pricing models offered by cloud providers, such as AWS’s Reserved Instances, Azure’s Savings Plans, or Google Cloud’s Committed Use Contracts. The AI solution can evaluate which pricing models provide the most cost-effective options for the organization’s unique usage patterns.
- Optimal Pricing Model Selection: By analyzing historical usage, AI can suggest a combination of on-demand, reserved, and spot instances to minimize costs while maintaining the necessary performance and availability levels.
- Discount Optimization: Cloud providers often offer volume discounts, promotional credits, or bundled services that can reduce costs. AI models can identify opportunities for applying these discounts and suggest the best approach for taking advantage of them.
Simulating Cost Optimization Scenarios
One of the most powerful capabilities of AI is its ability to simulate various cost optimization scenarios. By modeling different deployment strategies and configurations, AI can predict the impact on cloud spending over time. Cost implications of different choices, such as adjusting instance sizes, shifting workloads to different regions, or using alternative storage solutions can be understood by running simulations.
- What-If Analysis: AI-powered simulation tools allow enterprises to perform “what-if” analyses to understand the cost impact of potential changes in deployment strategies, such as shifting workloads from on-demand to reserved instances or using auto-scaling policies.
- Risk Management: Predictive models can assess the risk of committing to long-term savings plans, such as reserved instances or committed use contracts, based on projected future usage. This enables organizations to make informed decisions about cloud commitments.
Implementing Recommendations for a 3-6 Month Optimization Period
Using the insights gathered from AI-driven analysis, enterprises can implement a cloud cost optimization strategy over a 3-6 month period. The strategy may involve:
- Resizing or Rightsizing Resources: AI models can recommend resizing virtual machines (VMs), databases, and other cloud resources to better align with actual usage. This prevents over-provisioning and reduces unnecessary costs.
- Automating Resource Scheduling: AI can suggest scheduling policies to automatically start and stop non-essential workloads during off-peak hours which reduces costs associated with idle resources.
- Utilizing Spot Instances and Preemptible VMs: For non-critical workloads, AI can recommend leveraging spot instances (AWS) or preemptible VMs (Google Cloud) to reduce costs by up to 90%.
Monitoring and Continuous Improvement
AI-driven cloud cost optimization is not a one-time activity. To sustain savings, it is essential to continuously monitor cloud usage and adjust optimization strategies based on evolving needs. AI solutions can provide ongoing monitoring, detecting any cost deviations and automatically adjusting recommendations based on changes in usage patterns or pricing.
- Continuous Learning: ML algorithms can continuously learn from new data to refine cost optimization strategies. As usage patterns evolve, the AI models become more accurate in predicting optimal deployment configurations.
- Automated Alerts and Notifications: Enterprises can set up automated alerts which notify teams when costs exceed thresholds or when usage deviates from the norm. This enables proactive management of cloud spending.
Potential Savings: 30-40% Cost Reduction by the Third Month
The impact of AI-driven cloud cost optimization can be substantial, especially for enterprises with monthly cloud spending exceeding $10,000. Significant cost reduction can be achieved by following a structured approach to analyze usage, apply optimal pricing models, and implement AI-driven recommendations. In many cases, enterprises can expect to save 30-40% by the end of the third month.
- Case Study Example: An enterprise with a monthly cloud spend of $50,000 leveraged AI-powered optimization tools. Idle resources and rightsized instances were identified. By the third month, the company reduced its cloud costs by 38%, achieving annual savings of over $225,000.
Why Enterprises Need AI-Driven Cloud Cost Optimization
The benefits of AI-driven cloud cost optimization extend beyond cost savings. Here’s why enterprise CTOs, CIOs, and CFOs should prioritize this approach:
Conclusion
Managing cloud TCO is a complex and ongoing challenge for enterprises. Organizations can effectively optimize their cloud spending by leveraging Gen AI and ML. AI-driven tools provide a comprehensive approach to analyzing usage, optimizing pricing models, and implementing cost-saving strategies. For enterprises with significant cloud investments, this approach delivers substantial savings. This drives better financial visibility, operational efficiency, and strategic decision-making.
With AI-powered cloud cost optimization, enterprises can achieve smarter cloud spending and maintain a competitive edge in the ever-evolving digital landscape.
About the Author
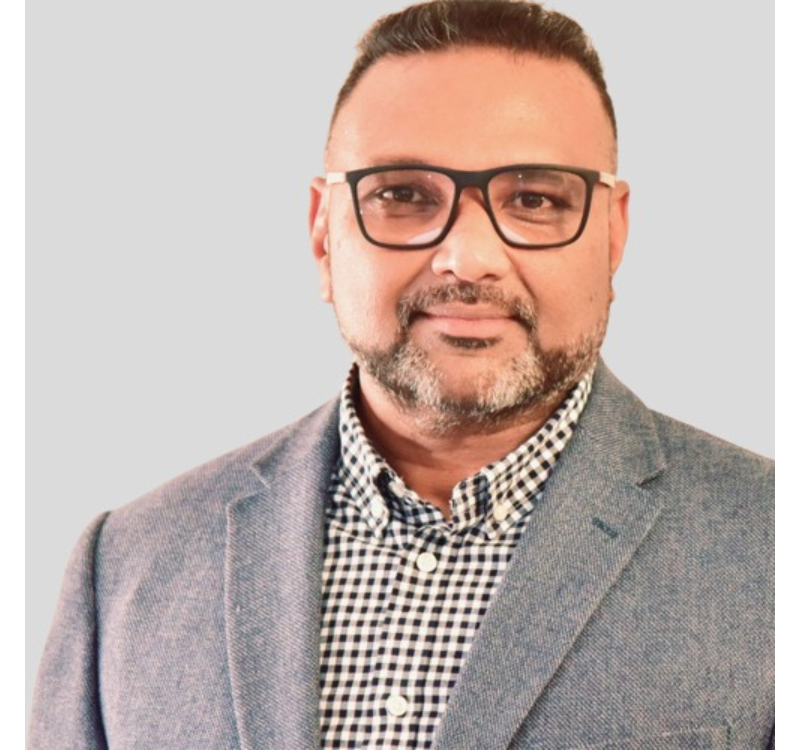
Rejith Krishnan
Rejith Krishnan is the Founder and CEO of lowtouch.ai, a platform dedicated to empowering enterprises with private, no-code AI agents. With expertise in Site Reliability Engineering (SRE), Kubernetes, and AI systems architecture, he is passionate about simplifying the adoption of AI-driven automation to transform business operations.
Rejith specializes in deploying Large Language Models (LLMs) and building intelligent agents that automate workflows, enhance customer experiences, and optimize IT processes, all while ensuring data privacy and security. His mission is to help businesses unlock the full potential of enterprise AI with seamless, scalable, and secure solutions that fit their unique needs.